Neuromorphology
Neuromorphology (from Greek νεῦρον, neuron, "nerve"; μορφή, morphé, "form"; -λογία, -logia, “study of”[1][2]) is the study of nervous system form, shape, and structure. The study involves looking at a particular part of the nervous system from a molecular and cellular level and connecting it to a physiological and anatomical point of view. The field also explores the communications and interactions within and between each specialized section of the nervous system. Morphology is distinct from morphogenesis. Morphology is the study of the shape and structure of biological organisms, while morphogenesis is the study of the biological development of the shape and structure of organisms. Therefore, neuromorphology focuses on the specifics of the structure of the nervous system and not the process by which the structure was developed. Neuromorphology and morphogenesis, while two different entities, are nonetheless closely linked.
History
Progress in defining the morphology of nerve cells has been slow in its development. It took nearly a century after the acceptance of the cell as the basic unit of life before researchers could agree upon the shape of a neuron. It was originally thought to be an independent globular corpuscle suspended along nerve fibers that looped and coiled.[3] It was not until the first successful microdissection of a whole nerve cell by Otto Deiters in 1865 that the separate dendrites and axon could be distinguished.[3] At the end of the 19th century, new techniques, such as Golgi's method, were developed that enabled researchers to view the whole neuron. This Golgi investigation then promoted new research in neuronal spacing by Ramon y Cajal in 1911. Further morphology research continued to develop, including dendritic morphology. In 1983 Thoroya Abdel-Maguid and David Bowsher expanded upon the golgi method and combined it with an impregnation technique which allowed them to visualize the dendrites of neurons and classify them based on their dendritic patterns.[4] Since then, myriad techniques have been developed and applied to the field of neuromorphology.
Influence on neuron function
Research has supported a relationship between the morphological and functional properties of neurons. For instance, the accordance between the morphology and the functional classes of cat retinal ganglion cells has been studied to show the relationship between neuron shape and function. Orientation sensitivity and dendritic branching patterns are a few other common characteristics of neurons that researchers have noted as having an effect on neuron function.[5] Ian A. Meinertzhagen et al. have recently established a connection between the genetic factors that underlie a specific neuronal structure and how these two factors then pertain to the neuron's function by examining the optic nerves in Drosophila melanogaster. They assert the structure of the neuron is able to determine its function by dictating synapse formation.[6]
The geometry of neurons often depends on the cell type and the history of received stimuli that is processed through the synapses. The shape of a neuron often directs the neuron's function by establishing its synaptic partnerships. However, there is also a growing evidence for volume transmission, a process that involves electrochemical interactions from the whole cell membrane.[5]
The axonal tree morphology is instrumental in activity modulation and information coding.[7]
Development
The development of the morphological features of neurons is governed by both intrinsic and extrinsic factors. The neuromorphology of nervous tissue is dependent upon genes and other factors, such as electric fields, ionic waves, and gravity. Developing cells additionally impose geometrical and physical constraints upon each other. These interactions affect the neural shape and synaptogenesis.[8] Morphological measures and imaging applications are important for further understanding the developmental process.
Subfields
General morphology
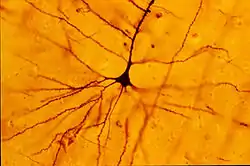
Since there is a broad range of functions performed by different types of neurons in diverse parts of the nervous system, there is a wide variety in the size, shape, and electrochemical properties of neurons. Neurons can be found in different shapes and sizes and can be classified based upon their morphology. The Italian scientist Camillo Golgi grouped neurons into type I and type II cells. Golgi type I neurons have long axons that can move signals over long distances, such as in Purkinje cells, whereas Golgi type II neurons generally have shorter axons, such as granule cells, or are anaxonic.[9]
Neurons can be morphologically characterized as unipolar, bipolar, or multipolar. Unipolar and pseudounipolar cells have only one process extending from the cell body. Bipolar cells have a two processes extending from the cell body and multipolar cells have three or more processes extending towards and away from the cell body.
Theoretical neuromorphology
Theoretical neuromorphology is a branch of neuromorphology focused on the mathematical description of the shape, structure and connectivity of the nervous system.
Gravitational neuromorphology
Gravitational neuromorphology studies the effects of altered gravity on the architecture of the central, peripheral, and autonomic nervous systems. This subfield aims to expand the current understanding of the adaptive capabilities of nervous systems, and specifically examines how environmental effects can alter nervous system structure and function. In this case environmental manipulations generally include exposing neurons to either hypergravity or microgravity. It is a subset of gravitational biology.[10]
Research methods and techniques
A variety of techniques have been used to study neuromorphology, including confocal microscopy, design-based stereology, neuron tracing[11] and neuron reconstruction. Current innovations and future research include virtual microscopy, automated stereology, cortical mapping, map guided automated neuron tracing, microwave techniques, and network analysis. Of the currently used techniques for studying neuromorphology, design-based stereology and confocal microscopy are the two most preferred methods. A complete database of neuronal morphology called the NeuroMorpho Database also exists.[12]
Design-based stereology
Design-based stereology is one of the most prominent methods for mathematically extrapolating a 3-D form from a given 2-D form. It is currently the leading technique in biomedical research for analyzing 3-D structures.[13] Design-based stereology is a newer stereology technique that examines morphology that has been predefined and designed. This technique contrasts with the older method, model-based stereology, which utilized previously determined models as a guide. The more current design-based stereology allows researchers to probe the morphology of neurons without having to make assumptions about their size, shape, orientation or distribution. Design-based stereology also gives researchers more freedom and flexibility as model-based stereology is only effective if the models are truly representative of the object being studied, while design-based stereology is not constrained in this way.[14]
Confocal microscopy
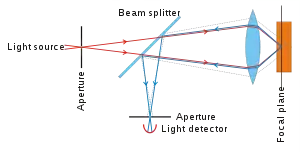
Confocal microscopy is the microscopic procedure of choice for examining neuron structures as it produces sharp images with improved resolution and decreased signal-to-noise ratio. The specific way this microscopy works allows one to look at one confocal plane at a time, which is optimal when viewing neuronal structures. Other more conventional forms of microscopy simply do not allow one to visualize all neuronal structures, especially those that are subcellular. Recently, some researchers have actually been combining design-based stereology and confocal microscopy to further their investigations into the specific neuronal cellular structures.
Cortical mapping
Cortical mapping is defined as the process of characterizing specific regions in the brain based on either anatomical or functional features. Current brain atlases are not definitive or homogenous enough to portray specific structural details. Recent advances in functional brain imaging and statistical analysis may however prove to be sufficient in the future. A recent development in this field called the Gray Level Index (GLI) method allows for more objective identification of cortical regions via algorithms. The GLI is a standardized method that allows researchers to determine neuron density. It is specifically defined as the ratio of area covered by Nissl-stained elements to area covered by unstained elements.[15] More sophisticated cortical mapping techniques are still in the process of being developed and this field will most likely see an exponential growth in mapping methods in the near future.
Clinical applications
Neuromorphology has been used as a new method of exploring the underlying cause of many neurological disorders, and has been included in the clinical study of various neurodegenerative diseases, mental disorders, learning disabilities, and dysfunctions due to brain damage. Researchers have been using neuromorphological techniques to not only study the damage but also ways to regenerate the damage nerve through ways like axon growth stimulation. Neuromophology has been used to study optical nerve damage, specifically looking at lesions and atrophies. Researchers have also examined and identified the neuromorphology of the human penis to better understand the role the sympathetic nervous system plays in achieving an erection. [16]
Current and future research
Computational neuromorphology
Computational neuromorophology examines neurons and their substructures by cutting them into slices and studying these different subsections. It also describes the neuromorphological space as a 3-D space. This allows researchers to understand the size of specific neuronal components. Additionally, the 3-D imaging helps researchers comprehend how the neuron transmits information within itself.[17]
Virtual microscopy
Virtual microscopy would allow researchers to obtain images with a decreased amount of imaging sessions, thus preserving the integrity of the tissue and decrease the possibility the fluorescent dyes fading during imaging. This method would additionally give researchers abilities to visualize currently unobtainable data, such as rare cell types and the spatial allocation of cells in a specific brain region.[13] Virtual microscopy would essentially allow for the digitization of all images obtained, therefore preventing deterioration of the data. This digitization could also potentially permit researchers to create a database to share and store their data.
References
- Morphology
- Neuron
- Peters, Alan; Palay, Sanford L.; Webster, Henry deF. (January 1991). The Fine Structure of the Nervous System: Neurons and Their Supporting Cells. New York: Oxford University Press. ISBN 978-0-19-506571-8.
- Abdel-Maguid, Thoroya; Bowsher, David (1984). "Classification of neurons by dendriticbranching pattern. A categorisation based on Golgi impregnation of spinal and cranial somatic and visceral afferent and efferent cells in the adult human". Journal of Anatomy. 138 (Pt 4): 689–702. PMC 1164353. PMID 6204961.
- Costa, Luciano da Fontoura; Campos, Andrea G.; Estrozi, Leandro F.; Rios-Filho, Luiz G.; Bosco, Alejandra (2000). "A Biologically-Motivated Approach to Image Representation and Its Application to Neuromorphology". Biologically Motivated Computer Vision. Lecture Notes in Computer Science. Vol. 1811. pp. 192–214. doi:10.1007/3-540-45482-9_41. ISBN 978-3-540-67560-0.
- Meinertzhagen, Ian A.; Takemura, Shin-ya; Lu, Zhiyuan; Huang, Songling; Gao, Shuying; Ting, Chun-Yuan; Lee, Chi-Hon (2009). "From Form to Function: the ways to know a neuron". Journal of Neurogenetics. 23 (1–2): 68–77. doi:10.1080/01677060802610604. PMID 19132600. S2CID 14625965.
- Ofer, Netanel; Shefi, Orit; Yaari, Gur (August 2017). "Branching morphology determines signal propagation dynamics in neurons". Scientific Reports. 7 (1): 8877. Bibcode:2017NatSR...7.8877O. doi:10.1038/s41598-017-09184-3. PMC 5567046. PMID 28827727.
- Costa, Luciano da Fontoura; Manoel, Edson Tadeu Monteiro; Fauchereau, Fabien; Chelly, Jamel; van Pelt, Jaap; Ramakers, Ger (July 2002), "A shape analysis framework for neuromorphometry", Network: Computation in Neural Systems, 13 (3): 283–310, doi:10.1088/0954-898x/13/3/303, PMID 12222815
- Purves, Dale; et al. (2001). Neuroscience (2. ed.). Sunderland: Sinauer Associates Inc. ISBN 978-0-87893-742-4.
- Krasnov, IB (December 1994). "Gravitational neuromorphology". Advanced Space Biology Medicine. 4: 85–110. doi:10.1016/s1569-2574(08)60136-7. PMID 7757255.
- Oztas, Emin (2003). "Neuronal tracing". Neuroanatomy. 2: 2–5.
- Costa, Luciano Da Fontoura; Zawadzki, Krissia; Miazaki, Mauro; Viana, Matheus P.; Taraskin, Sergei N. (December 2010). "Unveiling the Neuromorphological Space". Frontiers in Computational Neuroscience. 4: 150. doi:10.3389/fncom.2010.00150. PMC 3001740. PMID 21160547.
- Lemmens, Marijke A.M.; Steinbusch, Harry W.M.; Rutten, Bart P.F.; Schmitz, Christoph (2010). "Advanced microscopy techniques for quantitative analysis in neuromorphology and neuropathology research: current status and requirements for the future". Journal of Chemical Neuroanatomy. 40 (3): 199–209. doi:10.1016/j.jchemneu.2010.06.005. PMID 20600825. S2CID 178043.
- "What is design-based stereology". Retrieved 7 November 2011.
- Casanova, Manuel F.; Buxhoeveden, Daniel P.; Switala, Andrew E.; Roy, Emil (2002). "Neuronal Density and Architecture (Gray Level Index) in the Brains of Autistic Patients". Journal of Child Neurology. 17 (7): 515–21. doi:10.1177/088307380201700708. PMID 12269731. S2CID 25876423.
- Benson, George; McConnell, Joann; Lipshultz, Larry I.; Corriere, Joseph Jr.; Wood, Joe (1980). "Neuromorphology and Neuropharmacology of the Human Penis". Journal of Clinical Investigation. 65 (2): 506–513. doi:10.1172/JCI109694. PMC 371389. PMID 7356692.
- Trinidad, Pablo. "Computational Neuromorphology". University of Texas at Dallas. Archived from the original on 2 January 2009. Retrieved 2 November 2011.